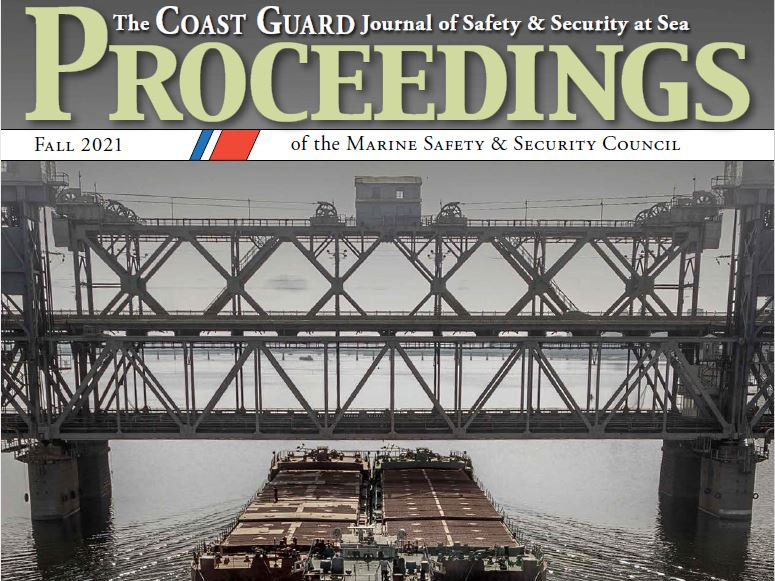
By Bill Keough, Anne Goodchild, and Giacomo Dalla Chiara
Consumers have become accustomed to having the products they order delivered faster than ever. Using innovative techniques to securely deliver goods wherever and whenever the customer requests them, delivery companies are advancing to meet this demand.
Competition throughout the supply chain is steadily growing. Companies need to devise innovative methods for the transportation of goods from raw materials all the way to the final consumer. From concept to practice, it can be challenging to identify affordable solutions.
This article will highlight recent research conducted by the University of Washington’s Urban Freight Lab. It will also detail work the lab has conducted with its strategic partners to explore new methods to reduce transportation costs, improve the customer experience, reduce carbon footprint, and reduce urban congestion after goods leave the shipping docks.
Customer Expectations in a World of ecommerce
Think of the first time you ordered something from Amazon, and the fulfillment experience when your product finally arrived. My first Amazon order in 1999 was for a newly released Tom Waits album. At the time I was working as a technology consultant for IBM and it seemed appropriate to try this out, especially since I was telling clients all about the business revolution internet commerce would soon create. I remember entering my credit card information with a little trepidation as I recalled an IBM security consultant telling me that he actually used a separate credit card for internet ordering due to the risks.
I liked the fact that Amazon immediately told me when my order would arrive. I think their projection was probably 5 days, which was fine since I didn’t need to do anything except be patient. I remember walking to my mailbox two days after placing the order and there was my CD. I guess Amazon’s thinking was to under-promise and over-deliver. It seems odd now, but I was really impressed.
That was a long time ago. Amazon now offers free delivery with its Prime program, and even two-hour delivery for some products in large metro areas. We all know how this has changed consumer expectations. As the pandemic began, I recall ordering a lawn spreader and I was particularly aggravated when Amazon indicated that I would need to wait a week to receive my item and I actually ended up buying it from Walmart because they could deliver in two days.
Consumers now expect to have an enormous catalog of products to choose from, and they expect the delivery to be on-time, in-full, undamaged, and with accurate documentation—a phenomenon called “perfect order.” On top of that, they also expect their deliveries to be free.
Although the pandemic has lengthened the amount of time consumers are willing to wait for a delivery, and increased their willingness to pay for expedited delivery, it seems reasonable that consumer expectations will return to pre-pandemic levels once the worst subsides. Adjusted consumer expectations during the COVID-19 outbreak have provided a brief respite for less capable ecommerce shippers, but this respite won’t last. Based on a number of research studies, delivery excellence is a key factor in a consumer’s perception of the brand. I know that I have stopped buying from merchants who were unable to meet their delivery commitments.
Contemporary Challenges in ecommerce Logistics
For most of us, when we shop online we expect to find exactly what we want—immediately available inventory, the most competitive prices, and complete, fast, free delivery. Amazon has taught us that this is what consumers should expect.
At this point in online retail, these abilities could almost be considered table stakes. But as any supply chain person will tell you, the operational, technological, and logistical challenges that are required to pull this off are gargantuan. Likewise, the pricing pressure exerted on logistics providers in this new competitive environment are unprecedented. To remain competitive, transportation providers must design and adopt innovative solutions that will fundamentally change the way products are delivered.
While logistics providers face a host of challenges in meeting these new service level requirements, one of the largest areas of opportunity is in optimizing delivery in what is termed the “last mile.” In logistics, the term last mile means the last step of the delivery process from a warehouse or distribution center to the end customer. Depending on the nature of the delivery, last mile transportation can actually encompass the 50 miles or more it takes to get the product to the final consumer’s door.
Last mile logistics is in fact the most expensive part of the delivery process. Most transportation providers are now focused on optimizing this part of the delivery process as new efficiencies can contribute very significantly to corporate profits, especially at a time when the shipping volumes and negotiating power of companies like Amazon and Walmart are exerting such downward pressure on shipping costs.
Going Beyond the Last Mile
Addressing the last mile problem is challenging. But the researchers at the Supply Chain Transportation & Logistics Center (SCTL) have analyzed this problem in depth and isolated what is, in fact, the most challenging and costly portion of the last mile—coining the term “The Final 50 Feet.”
The final 50 feet is the distance a package must travel to go from the delivery truck into the hands of the final consumer. For several years, the Urban Freight Lab at the University of Washington has been studying this problem and working with key stakeholders to devise, test, and implement innovative, real-world solutions to address what is probably the most daunting problem in the world of urban freight.
When I drive down the streets of downtown Seattle, the effects of the final 50 feet problem are painfully clear. As the number of deliveries being made downtown increases exponentially, Seattle’s already-challenging traffic situation is becoming untenable. The increasing traffic congestion is due in large part to trucks parked on the sidewalk, in alleys, or sometimes in the middle of the road.
The SCTL Urban Freight Lab (UFL) at the University of Washington has partnered with the Department of Energy, the Seattle Department of Transportation, and the Pacific Northwest National Laboratory (PNNL) on a pilot project to address the final 50 feet challenge. Our research considers leveraging contemporary sensor technology, machine learning, and new processes for accomplishing this delivery challenge.
Through the Department of Energy’s Office of Energy Efficiency and Renewable Energy, the Vehicle Technologies Office is funding the three-year, $1.5 million pilot project in Seattle and Bellevue, Washington, to:
- Reduce parking seeking behavior by approximately 20 percent in a pilot test area
- Reduce parcel truck dwell time by approximately 30 percent
- Increase business efficiency by increasing turnover in load/unload spaces
Project Approach
The UFL is taking a two-pronged approach by deploying centralized delivery lockers and collecting real-time data about parking behaviors and availability, then employing machine learning to predict the availability of parking at any moment in time. Both of these initiatives are focused on accelerating the delivery process to reduce urban congestion.
Accelerating the Delivery Process
Common carrier locker
The UFL partnered with the Seattle Department of Transportation to establish what are called common carrier delivery lockers in high-density population areas. Common carrier lockers are secure, automated, self-service storage systems designed to accommodate deliveries from multiple transportation providers delivering a range of parcel sizes. If you’ve ever had an Amazon package delivered to a locker you understand the idea. However, unlike Amazon lockers, these lockers are “common,” in that any carrier can use them to make deliveries. Such common carrier lockers create what is called delivery density, enabling many trucks to transport many packages to a single stop, rather than all those vehicles having to make multiple stops to accomplish the same task. This new approach reduces both dwell time and failed first deliveries, both of which produce congestion, unwanted emissions, and increased cost. Each is described below.
Reducing failed deliveries
Failed deliveries are a significant problem for delivery firms like UPS and DHL. A failed delivery occurs when the delivery driver is unable to get the package into the hands of the final consumer for any number of reasons. The most common reason for final delivery failure is that the consumer isn’t home and there is no safe place to leave the package so it doesn’t get stolen. Failed deliveries, which must be redelivered the next day, further increase congestion and pollution in urban areas. And they impact profits. Clearly, having to deliver a package twice instead of once makes that delivery that much less profitable.
Common carrier lockers essentially solve the failed delivery problem, not just for one carrier but for all of them. Fewer failed deliveries mean fewer delivery trucks downtown, so there’s less congestion and more available short-term parking. More parking means fewer delivery vehicles circling the block looking for somewhere to stop, or pulled up on the sidewalk.
Reducing dwell time
One of the important efficiency metrics for delivery vehicles is dwell time. Dwell time is the time that a vehicle spends at a scheduled stop without moving, in our case when the driver is making deliveries. Obviously, the shorter the dwell time the better. To quantify the benefits that this approach can provide, the UFL observed and timed actual urban deliveries. The conclusions were compelling. Using the traditional delivery approach without access to common carrier lockers, the average delivery time to deliver packages on seven different floors in a building in downtown Seattle was 27 minutes. With common carrier lockers, the average last 50-foot delivery time collapsed to only 5.6 minutes—a reduction of nearly 80 percent! Not surprisingly, this reduced truck dwell time by nearly the same amount. As a result, far more vehicles can use the same parking space, meaning fewer trucks blocking the alleys, on sidewalks, and less need for city planners to devise ways to create more truck parking.
This reduction not only reduces congestion, but it also enables delivery firms to conduct more profitable operations, since faster deliveries require fewer trucks and less labor. Property managers have also embraced this solution. It enhances building security since there are no longer unknown individuals inside the buildings they manage. It is also more environmentally friendly, as it saves fuel and reduces emissions.
Real-time Data Detection and Analysis
The first step in understanding the real-time availability of commercial parking accessible to delivery drivers is to know whether a parking space will be available when the driver arrives. There are a number of commercially available solutions for collecting this information, but most of them function in a similar manner. To support the considerable data integration, management, and analysis requirements of this effort, UFL has partnered with Pacific Northwest National Laboratory (PNNL).
A sensor that looks like a hockey puck is installed in the ground below a particular parking location. When a space becomes unoccupied, the sensor communicates this information to a vendor-specific data collection device. Depending on the device and the degree of connectivity, this data might either be stored on the collection device for a batch upload or streamed in real time. Ultimately, the data is sent to a server at the University of Washington and pushed to a server at PNNL through an application programming interface (API) for analysis using artificial intelligence capabilities built into the PNNL system.
The PNNL system will predict in real time which parking spots may be available when the driver arrives and where they are located, thereby dramatically reducing the amount of time drivers spend driving around looking for an available parking space. These sensors are relatively inexpensive, and the information they collect is binary—either a space is occupied or it is not. Obviously, gathering other data points besides vacancy status, like what type of vehicle is occupying the parking space at any given time, would be immensely useful in this effort, for example to ascertain the actual dimension of space occupied. However, at the current time only three data points—the location of the hockey puck and spaces, time of day, and vacancy status—are captured and communicated. Personally identifying information about people or vehicles is never gathered.
Rich Data
Several vendors have developed solutions that can provide additional data. Companies like Cleverciti and Verizon offer solutions that provide a wealth of additional data that is invaluable for this type of effort. These technologies capture and interpret far more detailed data about parking availability and usage.
These commercial solutions can capture real-time video of a particular urban location, stream that information to a server with software designed to interpret the video stream. They can also capture and analyze pedestrian traffic, automatically interpreting various attributes of the data it has collected. Likewise, such a system can be taught to recognize different types of delivery vehicles by color and shape, which companies the vehicles are affiliated with, what type of building they are visiting, license plate numbers, etc. Additionally, information about dwell times, occupancy, the routes vehicles take through a particular urban area at a particular time of the day, and other factors can be captured and analyzed. However, none of these data streams are made available to PNNL, only the occupancy status is provided through the API.
PNNL’s machine learning capabilities use only the parking space and occupancy history as model inputs to recognize patterns that are difficult for humans to discern, and then predict parking availability, based on the historical space occupancy information. Availability predictions will be accessible via a mobile device, enabling drivers to consider predicted parking availability in their decision making as they seek a suitable unoccupied parking spot. Even with these near real-time predictions, there are no guarantees a spot will remain open until the driver arrives to claim it. As the system learns, however, the predictions will increase in accuracy, delivering a far more powerful prediction solution.
Machine Learning
Once the occupancy data arrives at PNNL’s servers, the prediction models are generated, exploring parking behaviors across different parking spaces, as well as looking into parameters like the time of day and day of the week. Although it is beyond the project’s current scope, future improvements could explore contextual data sources, like weather or economic indicators, that influence congestion patterns to make even more intelligent decisions and projections.
Ultimately, this data will be available in a free mobile application that drivers can employ to locate parking locations that are currently empty.
The currently deployed version of the app allows drivers to define the length of their vehicle, view current occupancy, and determine if any applicable spaces are predicted to become available within a selected timeframe.
Future enhancements would be expected to improve the systems prediction accuracies. UFL member companies, like UPS, who will be using the app, will have the option to provide data like:
- vehicle length
- time of day when seeking parking
- the number of packages being delivered at each stop
- type of commodity being delivered—a single box or “big and heavy”
Drivers will also be able to select parking preferences. For example, a driver could indicate that they only want to be notified of spaces on the end of the block and not in the middle, because it is easier to maneuver.
To access the V2+ mobile application developed by PNNL, the users are required to provide an email address, which can be anonymous. Any additional data provided by the user to the application is used locally by the application and is not collected by or stored at PNNL. Such additional input data can direct drivers to available parking spots that meet their specific requirements. This information will also help drivers make more informed decisions about parking selection. In an ideal world, drivers with the app who are searching for a space would provide feedback if the space they were directed to is actually occupied at that particular moment. This is the sort of outcome-based data that helps the system “learn” to make more accurate predictions.
Other Outcomes
Removing waste and creating new business models and profit sources
While this research will unquestionably improve the efficiency and cost-effectiveness of delivery over the last 50 feet, this new real-time visibility will also create new business models and profit sources for resources that are currently either undervalued or entirely wasted. Similar examples of how technology is being employed to capture new value and remove waste in industries such as agriculture, where imperfect produce is now being sold to consumers rather than being discarded by farmers, is currently available.
Within the network of parking spaces available to delivery drivers, a significant percentage are privately-owned. At certain times these spots are vacant, even though there may be a lack of public parking options available to delivery drivers who may not want to pay for a space.
Once real-time information about parking accessibility is available, there is a foreseeable market for available private parking spots with prices that vary with demand, much like the way the price of an Uber fluctuates depending on demand.
Such a marketplace would remove waste from the system, creating a new profit-source for the owners of these private spaces while also providing a cost-effective solution for delivery drivers, who otherwise might be left to circle the block for 15 minutes seeking an available space.
Such innovations are what will contribute to the creation of “smart cities,” which deploy and integrate new technologies to improve urban services like utilities and transportation to reduce waste, the consumption of resources, and costs. In doing this, these innovations will also make cities more climate-friendly, more efficient, and, ultimately, more livable.
About the authors:
Bill Keough is the managing director of the Supply Chain Transportation and Logistics master’s program at the University of Washington. He also teaches in the program. His 20 years of consulting experience includes work at IBM, CSC, and with his own supply chain consulting firm, Keough Associates, helping private equity firms improve the performance of their portfolio companies. Mr. Keough holds degrees from Cornell University and the University of Houston.
Anne Goodchild is a professor of civil and environmental engineering at the University of Washington, and serves as founding director of both the supply chain transportation and logistics online master’s degree program and the Supply Chain Transportation & Logistics Center. The latter is home to the Urban Freight Lab, a public-private research consortium. She holds both a doctorate and a master’s degree from University of California, Berkeley, and a bachelor’s degree from University of California, Davis.
Giacomo Dalla Chiara, Ph.D. is a postdoctoral research associate at the University of Washington’s Urban Freight Lab. Before moving to Seattle, he was a research fellow at the Singapore University of Technology and Design in 2018 and a visiting scholar at the Massachusetts Institute of Technology in 2017. He holds a Ph.D. in engineering systems from the Singapore University of Technology and Design, a M.Sc. in statistics from ETH Zurich in Switzerland, and a B.Sc. in economics from LUISS University in Italy.
References:
Goodchild, A., Kim, H., & Ivanov, B. Final 50 Feet of the Urban Goods Delivery System: Pilot Test of an Innovative Improvement Strategy. (2019).
Urban Freight Lab. The Final 50 Feet Urban Goods Delivery System; Research Scan and Data Collection Project. (2018).